May 23, 2018 at 02:02PM
via New on MIT Technology Review
Machine vision technology has revolutionised the way we see the world. Machines now outperform humans in tasks such as facial recognition and many types of object recognition. And this technology is now employed in a wide range of applications from security systems to self-driving vehicles.
But there are still areas where machine vision techniques have yet to make such a strong impact. One of them is in analysing satellite images of the Earth. That’s something of a surprise since satellite images are numerous, relatively consistent in the way they are taken and crammed full of data of one kind or another. They are ideal for machines.
And yet, most satellite image analysis is done by human experts trained to recognise relatively obvious things such as roads, buildings and the way land is used.
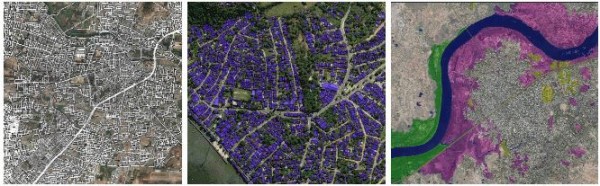
That looks set to change with the DeepGlobe Challenge organised by researchers at Facebook, the satellite imagery company, DigitalGlobe along with some academic partners at universities including MIT.
The challenge is to use machine vision techniques to automate the process of satellite image analysis. The results of the challenge are due to be announced next month.
The challenge has been clearly defined by the DeepGlobal team. The goal is to find automated ways to identify three types of information in satellite images: road networks, buildings and to determine land use. So the task is take an image as an input and to produce as an output one of the following: a mask showing the road network; an overlaid set of polygons representing buildings; or a colour-coded map showing land use, ie whether for agriculture, urban use, forest and so on.
For each of these three tasks, researchers have created a database of annotated images that entrants can use to train their machine vision systems. The entrants must then test their machine vision system on a test database to see how well it does.
The datasets are comprehensive. The road identification dataset includes some 9000 images with a ground resolution of 50cm, spanning a total area of over 2000 square kilometres in Thailand, Indonesia and India. The images include urban and rural areas with paved and unpaved roads. The training dataset also includes a mask for each image showing the road network in that area.
The buildings dataset includes over 24,000 images, each showing a 200 metre by 200 metre area of land in Las Vegas, Paris, Khartoum and Shanghai. The training dataset contains over 300,000 buildings, each one marked by human experts as an overlaid polygon.
The land use dataset consists of more than 1000 RGB images with 50 cm resolution paired with a mask showing land use as determined by human experts. The uses include urban, agriculture, rangeland, forest, water, barren and unknown (ie covered by cloud).
The DeepGlobe Challenge team have developed a number of algorithms for measuring the accuracy of machine-generated data that they can use to assess each of the entrants.
And there have been plenty of them: some 950 teams have registered to take part. The winners will be announced at a conference in Salt Lake City on 18 June.
There looks to be plenty of low-hanging fruit here. The major benefits are likely to be for people in remote areas that have not yet had their road networks mapped. One of the sponsors of the challenge is Uber, which may be able to use this type of data to extend its services.
It should also be useful when natural disasters strike and emergency services must reach and explore these areas quickly.
Made widely available at low cost, this data could also be useful for climate change research and for urban planning. And that should just be the beginning. This kind of analysis is surely just a stepping stone to a more detailed understanding the world around us. It’ll be interesting to see how well the participants perform.
Ref: arxiv.org/abs/1805.06561 : DeepGlobe 2018: A Challenge to Parse the Earth through Satellite Images